The importance of automated detection, tracking, classification and identification (DTCI) of military assets is growing rapidly. This evolution has a direct influence on military operations relying on conventional camouflage techniques, as the probability of camouflaged object detection increases with improved DTCI solutions. Therefore, the need for innovative camouflage solutions is growing. This is sharply underlined by the figure on the left, taken on April 1st 2022, when Belgian Defence proudly presented its new future camouflage clothing. The bounding boxes on the figure present the result of the famous YOLO5 detector on this image and clearly show that – in the ‘eyes’ of the AI – the military soldiers are as clearly visible (similar confidence level) as the MoD, meaning that the obfuscation effect of the military clothing is totally negligible for the AI.
ARC will remedy this situation by proposing new camouflage designs that will better protect soldiers and equipment against detection by enemy AI tools.
In a first phase of the study, the researcher will focus on visual sensing and investigate the static use case, developing techniques for the obfuscation of non-moving targets. A state-of-the-art study of DTCI AI techniques will be performed to highlight the main techniques that are in use (currently and expected in the near future) on the battlefield. For each of these techniques, the network architecture will be analyzed to find weaknesses in the DTCI accuracy that could be exploited. The main focus will be on concealed object detection AI techniques, as camouflaged object tracking, classification and identification rely on a detection phase. Based on this, physical obfuscation mechanisms (e.g. prints) will be proposed and field-tested under varying illumination conditions.
In a second phase, the study will investigate the dynamic use case, as multiple threat detection tools make use of the analysis of motion patterns for discerning between different types of objects (a typical example are DTCI AI solutions that detect the human gait or that target drones, while discerning birds). Similar to the first use case, weaknesses in the DTCI network architecture will be sought in order to propose novel camouflage for mobile ground, aerial or maritime systems.
In a third phase, the research will be extended to the non-visual domain. It is obviously impossible to incorporate an in-depth investigation over all possible sensing methodologies. Therefore, we will focus in the first place on thermal imaging as an extra sensor and assess in close collaboration with the military end users whether it is useful to integrate even more perception tools.
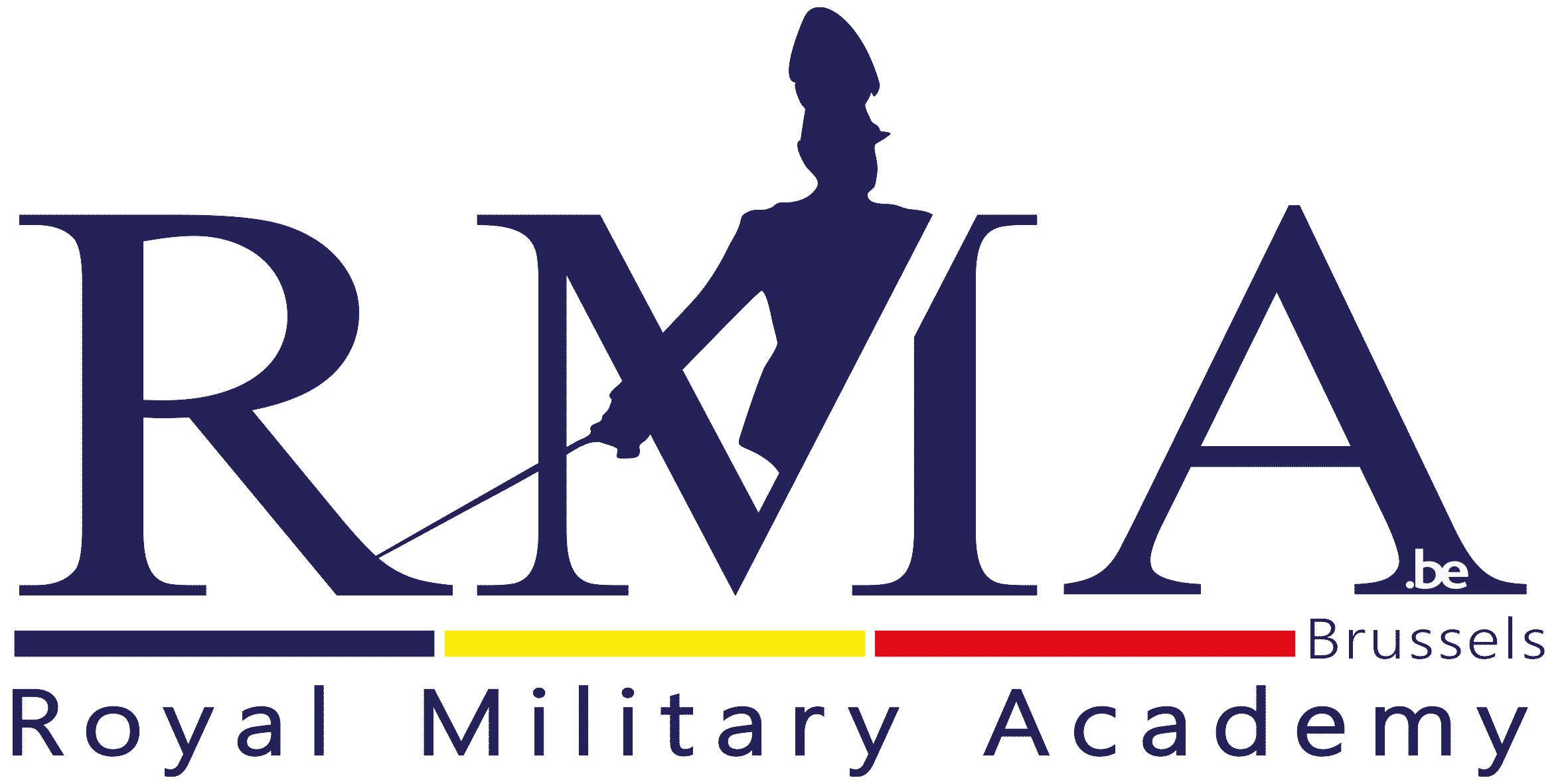
Project Publications
2025
- K. Cools, C. Maathuis, G. {De cubber}, M. Vandewal, and N. Deligiannis, “Evaluation Techniques for Modern Military Camouflage," in Evaluation Techniques for Modern Military Camouflage, 2025.
[BibTeX] [Download PDF]@inproceedings{0a1d528b681b4dc7a81b6b8487df795e, title = "Evaluation Techniques for Modern Military Camouflage", author = "Kasper Cools and Clara Maathuis and {De cubber}, Geert and Marijke Vandewal and Nikos Deligiannis", year = "2025", language = "Nederlands", booktitle = "Evaluation Techniques for Modern Military Camouflage", note = "International Conference on Military Technologies 2025, ICMT2025 ; Conference date: 27-05-2025 Through 30-05-2025", url = "https://icmt2025.cz", unit= {meca-ras}, project= {ARC} }
- C. Maathuis and K. Cools, “FHATMO: Feedback Model for Human-AI Teaming in Military Operations," in FHATMO: Feedback Model for Human-AI Teaming in Military Operations, 2025.
[BibTeX] [Download PDF]@inproceedings{7b6f43681fff433b80174d2a190df291, title = "FHATMO: Feedback Model for Human-AI Teaming in Military Operations", author = "Clara Maathuis and Kasper Cools", year = "2025", language = "Nederlands", booktitle = "FHATMO: Feedback Model for Human-AI Teaming in Military Operations", note = "International Conference on Military Technologies 2025, ICMT2025 ; Conference date: 27-05-2025 Through 30-05-2025", url = "https://icmt2025.cz", unit= {meca-ras}, project= {ARC} }
- C. Maathuis and K. Cools, “Risks and Control Measures for Building Trustworthy Autonomous Weapon Systems," in ICCWS – 20th International Conference on Cyber Warfare and Security, 2025.
[BibTeX]@inproceedings{8087a20d04e3490ca837aa9b8f5d158c, title = "Risks and Control Measures for Building Trustworthy Autonomous Weapon Systems", author = "Clara Maathuis and Kasper Cools", year = "2025", language = "Nederlands", booktitle = "ICCWS - 20th International Conference on Cyber Warfare and Security", unit= {meca-ras}, project= {ARC} }
- C. Maathuis and K. Cools, “The Role of AI in Military Cyber Security: Data Insights and Evaluation Methods," in The Role of AI in Military Cyber Security: Data Insights and Evaluation Methods, 2025, p. 191–200.
[BibTeX] [DOI]@inproceedings{2cd3f64017734a629399671997131f32, title = "The Role of AI in Military Cyber Security: Data Insights and Evaluation Methods", author = "Clara Maathuis and Kasper Cools", year = "2025", doi = "10.1016/j.procs.2025.02.078", language = "English", pages = "191--200", booktitle = "The Role of AI in Military Cyber Security: Data Insights and Evaluation Methods", publisher = "Elsevier Science Publishers B.V.", unit= {meca-ras}, project= {ARC} }
2024
- K. Cools and C. Maathuis, “Trust or Bust: Ensuring Trustworthiness in Autonomous Weapon Systems," in IEEE Military Communication Conference 2024, 2024.
[BibTeX] [Download PDF] [DOI]@inproceedings{b0d1d2ad7c7446dfbe873b15d4b3b337, title = "Trust or Bust: Ensuring Trustworthiness in Autonomous Weapon Systems", author = "Kasper Cools and Clara Maathuis", year = "2024", month = dec, doi = "10.1109/MILCOM61039.2024.10773908", language = "English", booktitle = "IEEE Military Communication Conference 2024", note = "2024 IEEE Military Communications Conference ; Conference date: 28-10-2024 Through 01-11-2024", url = "https://milcom2024.ieee-milcom.org/", unit= {meca-ras}, project= {ARC} }
2012
- G. De Cubber, D. Doroftei, Y. Baudoin, D. Serrano, K. Chintamani, R. Sabino, and S. Ourevitch, “ICARUS: AN EU-FP7 PROJECT PROVIDING UNMANNED SEARCH AND RESCUE TOOLS," in IROS2012 Workshop on Robots and Sensors integration in future rescue INformation system (ROSIN’12), Villamoura, Portugal, 2012.
[BibTeX] [Abstract] [Download PDF]
Overview of the objectives of the ICARUS project
@InProceedings{de2012icarus02, author = {De Cubber, Geert and Doroftei, Daniela and Baudoin, Y and Serrano, D and Chintamani, K and Sabino, R and Ourevitch, S}, booktitle = {IROS2012 Workshop on Robots and Sensors integration in future rescue INformation system (ROSIN'12)}, title = {{ICARUS}: AN {EU-FP7} PROJECT PROVIDING UNMANNED SEARCH AND RESCUE TOOLS}, year = {2012}, abstract = {Overview of the objectives of the ICARUS project}, project = {ICARUS}, address = {Villamoura, Portugal}, url = {http://mecatron.rma.ac.be/pub/2012/Icarus - ROSIN2012 Presentation.pdf}, unit= {meca-ras} }